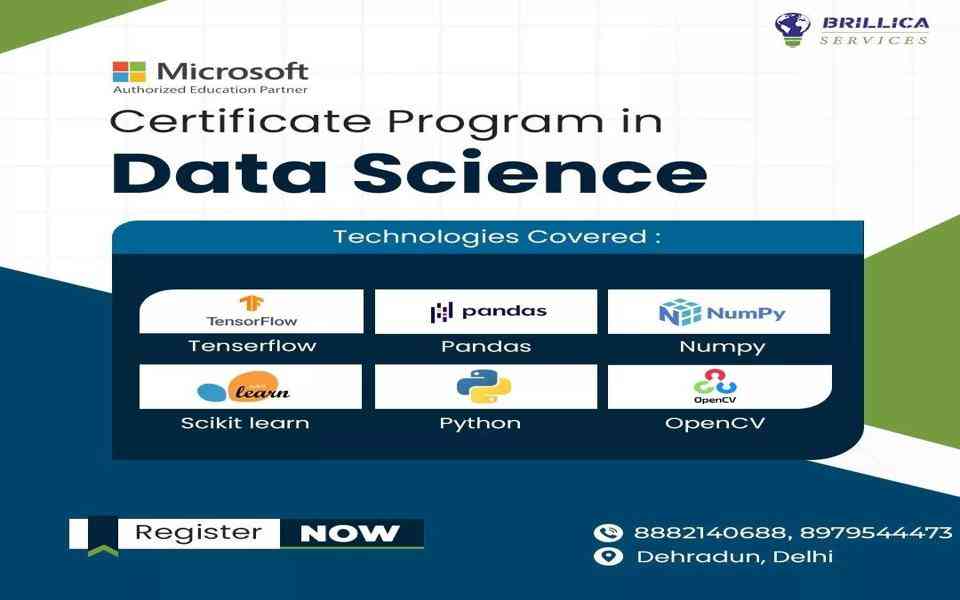
Basics of Tableau
Learn the basics of Tableau! Discover how to visualize data, create interactive dashboards, and gain insights effortlessly.
© 2024 Crivva - Business Promotion. All rights reserved.