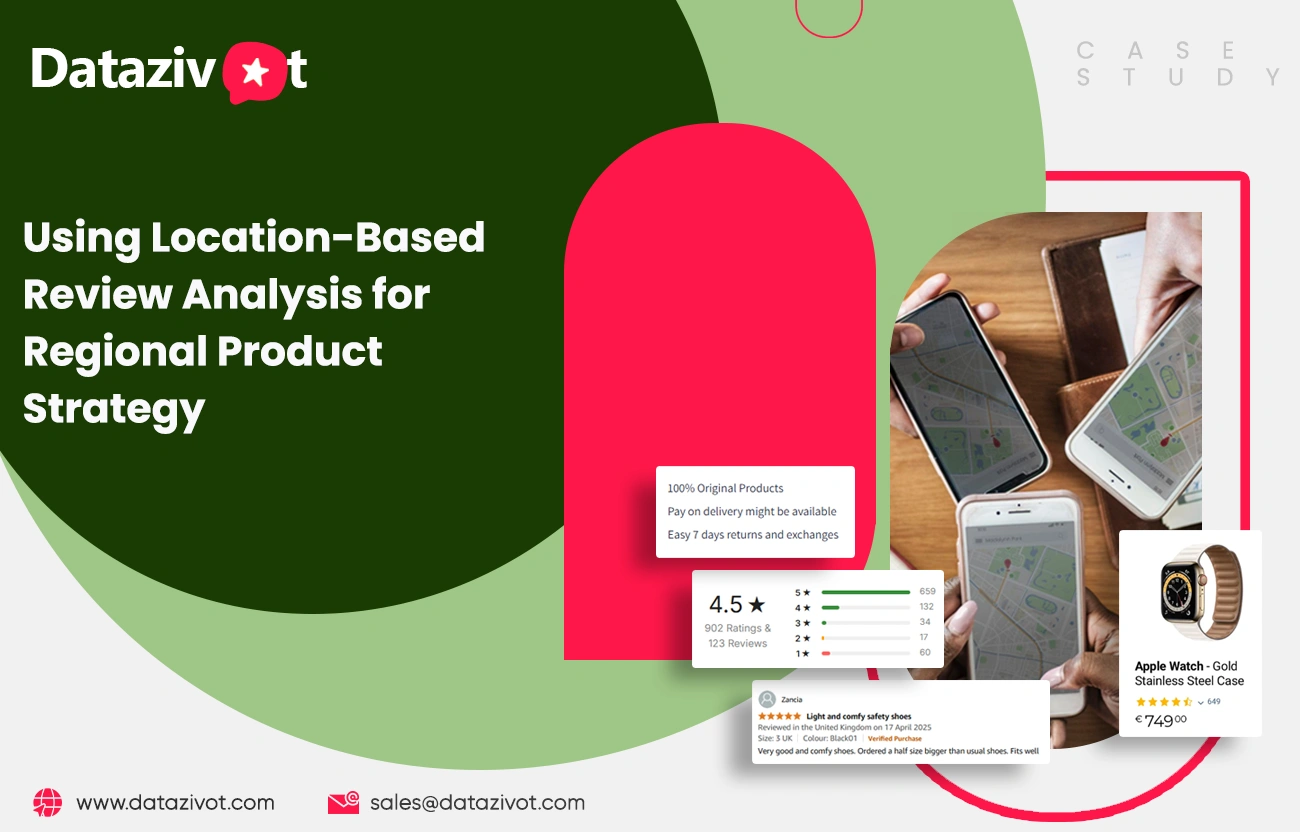
Business Challenge
A nationwide consumer brand was seeing mixed sales performance across different states.
They struggled to understand:
- Why certain products performed better in Bangalore vs Lucknow
- Why return rates were higher in Hyderabad than in Delhi
- What regional preferences existed across platforms like Amazon, Flipkart, and Myntra
“We’re selling the same SKU in every zone — but customers are reacting differently. We need data to localize better.”
They partnered with Datazivot to analyze location-tagged reviews and create a regional product strategy backed by sentiment and behavioral insights.
Objectives
- Scrape reviews from Amazon, Flipkart, and Myntra with geo-tags (explicit or inferred).
- Map sentiment trends by city/state.
- Identify regional preferences and pain points for products.
- Help the brand localize campaigns, inventories, and messaging.
Our Approach
1. Location-Aware Review Scraping
We extracted:
- Explicit city mentions in reviewer profiles (Amazon/Flipkart)
- Indirect location clues (language, delivery references: “Bangalore weather,” “Ahmedabad store”)
- Platform-level region filters for category listings
Platforms covered:
- Amazon.in – Electronics & personal care
- Flipkart – Fashion, FMCG, phones
- Myntra – Apparel and footwear
Review dataset: 450K+Review Datasets, 120+ cities covered
2. City-Wise Sentiment & Keyword Analysis
We broke down reviews by:
- Region → City → Pin-code clusters
- Product category → Subcategory
- Sentiment distribution (positive, neutral, negative)
- Keyword trends by region
Example mapping:
“Sweatproof,” “slim-fit,” “bright color,” “festive” reviews in South India
“Warm fabric,” “true size,” “pale tone” in North India winters
Sample Regional Insights Table
3. Geo-Sentiment Dashboards
We developed city-wise dashboards with:
- Top SKUs and review performance per region
- Keyword cloud and pain point mapping
- Negative review heatmap by pin-code zone
- Comparison across platforms (e.g., Amazon in Chennai vs Flipkart in Chennai)
Results & Strategic Actions
1. Localized Inventory Allocation
- Bangalore, Chennai, Hyderabad preferred lighter fabrics and breathable designs.
- Stocking of heavy-knit ethnic wear reduced in South India during Q3, saving ~₹14 lakh in overstock costs.
Reviews told the brand what wasn’t working in each region—before sales drop made it obvious.
2. Hyperlocal Campaign Personalization
- Flipkart reviews in Indore and Jaipur showed strong sentiment for “festive look,” “vibrant colors,” and “traditional embroidery.”
- Ads for those zones began using regional influencers and ethnic hooks.
Result: 22% CTR increase and 15% conversion lift in Tier-2 festive season campaigns.
3. Return Rate Reduction
- In Hyderabad, 1 in 4 negative reviews for shoes involved “tight fit.”
- Sentiment dashboard flagged this in real-time, leading to immediate fit chart customization and additional size availability in the region.
Return rates in Hyderabad dropped from 21% → 13% in 45 days.
Visual: Geo-Sentiment Heatmap (Sneakers Category)
Stack Used
Tool | Use Case |
---|---|
Scrapy + Proxies | Geo-tagged review scraping |
NLTK + spaCy | Location/entity extraction |
BERT | Review sentiment classification |
GeoPandas + Mapbox | City/pincode-level heatmaps |
Power BI | Interactive dashboard for brand teams |
Strategic Takeaways
Regional review analysis gave brand teams city-specific clarity.
- Campaigns could now match cultural preferences and pain points.
- Inventory was better optimized, reducing reverse logistics costs.
- Marketing & supply chain teams finally had shared real-time location insights.
Conclusion
Datazivot helped transform regional guesswork into data-driven product and campaign decisions.
Location isn’t just a shipping detail — it’s a powerful signal of what your customer values.